Transforming Machine Learning with Advanced Data Labeling Techniques
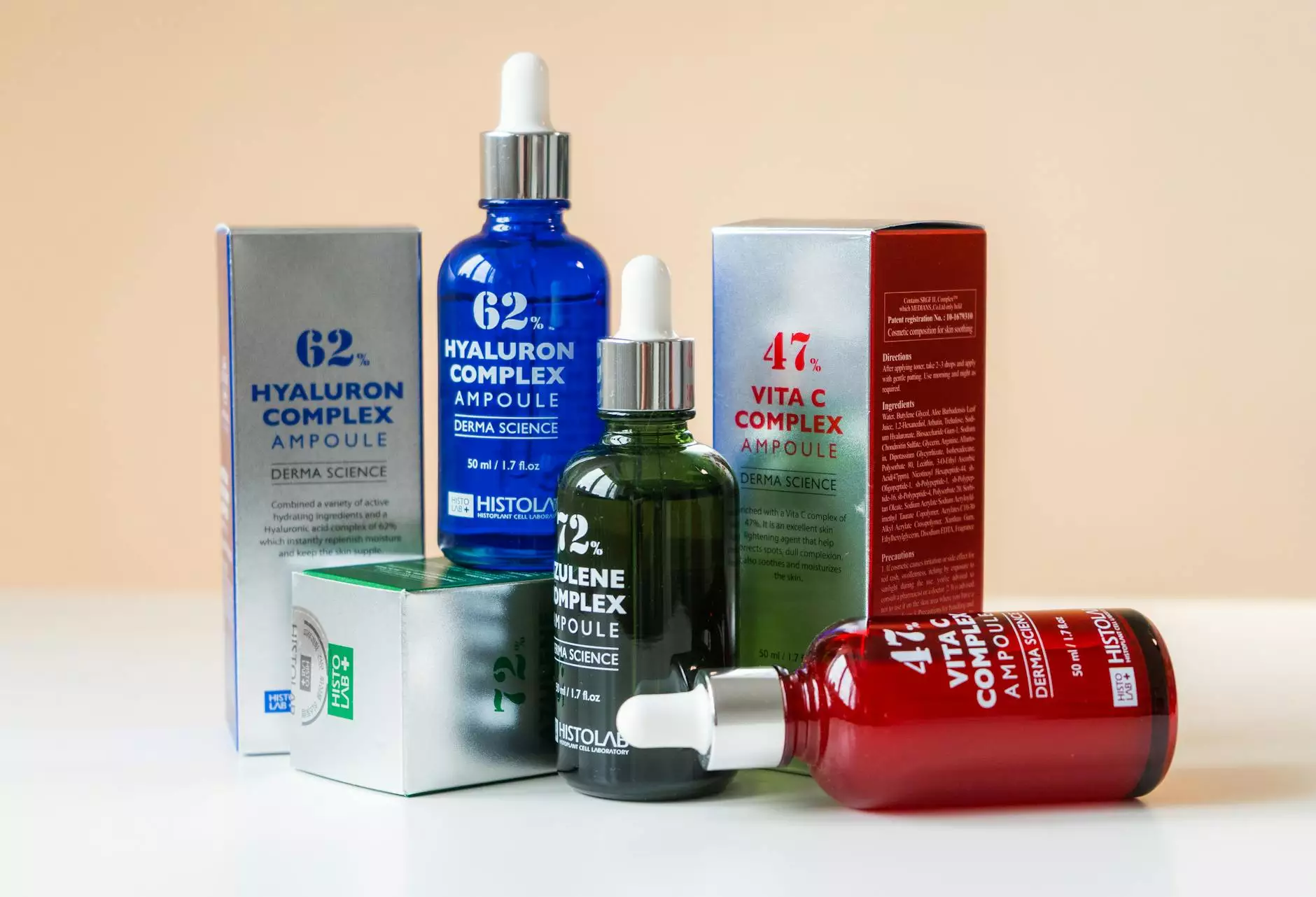
Understanding the Importance of Data Labeling in Machine Learning
In the realm of machine learning, data is the cornerstone of innovation and predictive modeling. However, raw data lacks the contextual meaning that machines need to learn effectively. This is where data labeling becomes crucial. Data labeling is the process of tagging data with meaningful labels that can provide context to algorithms, thereby enhancing their learning capacity. Businesses investing in robust data annotation solutions, such as those offered by KeyLabs.ai, are positioned for success in this competitive landscape.
What is Data Labeling?
Data labeling involves attaching metadata to various types of data (text, images, videos, etc.) to create a structured dataset for machine learning algorithms. It enables computers to interpret the data akin to human understanding. This not only facilitates effective model training but also improves accuracy in predictions during deployment.
Types of Data Labeling
- Image Annotation: Used in self-driving cars and facial recognition systems.
- Text Annotation: Essential for natural language processing tasks such as sentiment analysis and chatbots.
- Audio Annotation: Vital for speech recognition and audio classification tasks.
- Video Annotation: Important for action recognition and object detection in videos.
Why is Data Labeling Crucial for Machine Learning Success?
The efficiency of machine learning models hinges on the quality of the training data. Here are a few reasons why effective data labeling is indispensable:
1. Improved Model Accuracy
High-quality labeled data leads to superior model performance. When models are trained on accurately labeled datasets, they can achieve higher rates of precision, recall, and overall accuracy in their output. This is especially critical in applications like healthcare and finance, where errors can have severe repercussions.
2. Enhanced Training Speed
Well-annotated data accelerates the training process. When the data is neatly classified, machine learning algorithms can quickly assimilate the relationships and patterns without wasting resources on deciphering unstructured data. KeyLabs.ai offers rapid data annotation services that can significantly reduce training time.
3. Contextual Insight for AI
AI systems mimic human-like decision-making, which requires understanding context. Data labeling provides this context, enabling machines to make informed predictions. For example, an email filtering system relies heavily on labeled data to distinguish between spam and legitimate messages, ensuring efficient mail management.
KeyLabs.ai: Pioneering Data Annotation Tools and Platforms
As the demand for structured data continues to rise, KeyLabs.ai stands out as a leading provider of advanced data annotation tools and platforms. Our innovative platform streamlines the data labeling machine learning process and enhances productivity through automation and accuracy.
Features of KeyLabs.ai Data Annotation Platform
- Machine Learning Integration: Our tools leverage machine learning algorithms to automate parts of the annotation process, reducing manual effort while maintaining high accuracy.
- User-Friendly Interface: An intuitive interface ensures that both novices and experts can easily navigate the platform and complete projects efficiently.
- Quality Assurance Mechanisms: Built-in quality control features verify the accuracy of labels, ensuring high-quality data for model training.
- Scalability: Our platform can handle projects of any scale, accommodating the varying needs of businesses, from startups to enterprises.
- Customizability: Flexible labeling options cater to specific industry requirements, whether in healthcare, finance, retail, or any other sector.
Best Practices for Effective Data Labeling
To achieve optimal results in data labeling for machine learning, businesses should adhere to certain best practices:
1. Define Clear Objectives
Before initiating the data labeling process, it is essential to establish clear objectives. Understand what the model is expected to accomplish and how labeled data will enhance its performance. This clarity guides the labeling efforts and ensures alignment with the end goals.
2. Invest in Professional Annotation Services
While it might be tempting to handle data labeling in-house, investing in professional services like those offered by KeyLabs.ai often yields better results. Experts possess the experience and tools necessary to provide high-quality annotations, preventing costly delays and model inaccuracies related to poor data quality.
3. Regularly Review and Update Labels
Machine learning models evolve, and so should the data used to train them. Regular reviews of labeled data ensure that it remains relevant and accurate. This iterative process is essential for maintaining model performance over time and adapting to new trends or data types.
Challenges in Data Labeling
Despite its importance, data labeling is not without challenges. Some of the common obstacles include:
1. Labor-Intensive Process
Data labeling can be a time-consuming and resource-heavy activity, especially with large datasets. Choosing the right tools and platforms, such as KeyLabs.ai, can alleviate this burden by automating parts of the process and streamlining workflows.
2. Ensuring Consistency and Quality
Maintaining consistency in labeling is crucial for model training. Inconsistent labels can confuse algorithms and lead to poor learning outcomes. Implementing quality assurance processes and using experienced annotators can mitigate this risk.
3. Handling Ambiguities in Data
Data can often be ambiguous, requiring human judgment to interpret correctly. This subjectivity can lead to discrepancies in labeling. By leveraging collaborative annotation tools that allow team input, the risk of mislabeling can be reduced.
The Future of Data Labeling in Machine Learning
As machine learning continues its rapid evolution, the demand for precise data labeling will only increase. The future holds exciting possibilities, such as:
1. Autonomous Annotation Systems
The integration of AI and machine learning in the annotation process will likely lead to more autonomous systems capable of labeling data with minimal human intervention. This evolution will significantly enhance efficiency and reduce costs.
2. Crowd-Sourced Labeling Solutions
Platforms that facilitate crowd-sourced labeling can tap into a vast human resource for data annotation, ensuring diversity in inputs and speeding up the process. This collaborative approach can result in high-quality labeled datasets that reflect varied perspectives and minimize bias.
3. Integration with Real-Time Data Streams
As businesses leverage real-time data for decision-making, the ability to annotate data in real time will become increasingly important. Future platforms may provide tools for instantaneous labeling as data is ingested, enhancing the agility of machine learning models.
Conclusion
In conclusion, data labeling machine learning is an essential component of the machine learning ecosystem. As technology advances, companies that prioritize effective data annotation practices will stay ahead of the competition. By utilizing platforms like KeyLabs.ai, businesses can ensure they have access to high-quality labeled datasets that drive innovation and empower their machine learning initiatives.
For more information on how KeyLabs.ai can assist your business with its data annotation needs, visit KeyLabs.ai today.